Learn how vision systems for robotics can benefit your production logistics processes, discuss the trends and challenges associated with the technology, and how it enhances digital transformation
Robot vision enables a wide variety of improvements for production logistics. Applications often include bin picking, quality control, and robot guidance. With the many technological advances that digital transformation brings, it also generates new trends, and creates new challenges for robot vision use and applications.
One of the applications for robot vision is to ensure quality in production logistics. This ability complements Industry 4.0, which strives to achieve more efficiency and optimize processes through data. Vision in robotic systems provides quality in two main ways: inspection and predictability. Inspection applications directly impact quality through operating on the item and detecting defects. Predictability indirectly impacts quality in many ways that often need interpretation by a human.
These play into the I4.0 initiative in a few ways. They are identifying and flagging defective products which allows the robot to interact with the process more effectively. Additionally, as these vision systems are operating, they can also be recording and uploading data obtained to an external system. The data can be used to better understand trends in deficiencies and allow for a faster and more effective response to the deficiencies. In the case of deep learning, this data can also be used to continually improve the model.
AI and Machine Vision Play a Role
AI and Machine Vision are part of the robotics machine vision field. Machine vision, which is a form of artificial intelligence, is very prominent in robotics today. It is valued in the tens of billions in USD, when considering all applications. The pandemic has made it an even more intriguing field as end users look to create more automated and flexible processes due to labor shortages.
There are both mature and emerging areas to the robotic machine vision field. On one hand, there are traditional rule-based algorithms like pattern matching, OCR, and other tools which have been allowing robots to complete pick-and-place and inspection tasks for decades. On the other hand, there are machine learning and deep learning applications that are allowing the industry to complete tasks that seemed impossible a few years ago like anomaly detection in wood grains.
The mature, rule-based products are prominent in the robotic machine vision field. At least a few can be found in just about every facility using robotics. They have also been developed enough to make them easy to use and highly reliable. SICK’s PLB system is one example of this. It is a reliable and safe solution for robotics applications.
In contrast, machine learning and deep learning are just getting started in vision robotics. Due to complexity and need for further development, they are currently reserved for applications where they are absolutely needed over their traditional rule-based vision counterparts. They receive a lot of attention though because they have the potential to alter robotics as we know them. The “Gartner Hype Curve for Artificial Intelligence 2020” places deep learning at a two-to-five-year timeframe for general productive use.
Main Challenge: Environmental Impact
The environment the machine lives in can have an impact on a vision system. And it is one of the main challenges for the past decade and continues to be one. If this impact did not exist, many other challenges would vanish. Many vision problems arise from the combination of inadequate vision lighting (often to save on cost or space) and changes in ambient light (from an open bay door, skylight, or environmentally friendly lighting). It causes the features in the image to change, particularly in contrast, which can cause the vision tools to error unexpectedly. It can often be difficult to fix with the programming of the vision system alone causing headaches for everyone involved.
It is a good lighting solution for a vision system when the lights can be on or off and the image looks the same. This means that all ambient light is effectively drown out. It is a very simple thought, but one that should be stressed: good lighting makes for robust robotic vision systems.
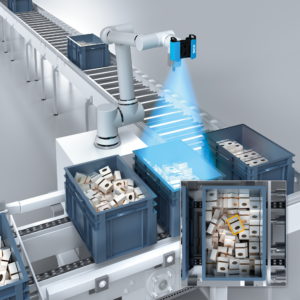
Industry Changes and Trends
The biggest change in the robotics industry in recent years is the adoption rate of small and medium end users. In the past, robotic applications were generally reserved for large end users with experience in technical resources such as those in the automotive and electronics spaces with high-volume-low-mix applications.
Today, new technology has enabled more flexible and easier to use systems including vision systems. This has led to small and medium end users, those with high-mix-low-volume and less experienced technical resources, to automate processes with robots.
Trends in the vision robotics field come down to two words: simplify complexity. End users are attempting more complex vision applications but want to simplify the way they are built, programmed, and supported. Many small to medium sized end users also may want to DIY the integration to cut costs. This has led to a rise in more configurable and “no-code” solutions. These solutions allow users to build complex applications without advanced knowledge of robotics or machine design.
Simplifying the Complex
Think of an application involving a complex task like bin picking or deep learning. Just a few years ago, these types of applications were considered far too complex for practical use like in production or a warehouse. Today, companies have developed products, like SICK’s PLB or dCloud, to simplify how the complex tasks are completed. These products have opened the market for these complex applications.
For instance, SICK’s PLB software allows a user to solve a bin picking application in a few configurable steps and have their robot picking parts within a couple of hours of unboxing the camera. Older technology, such as 2D vision, is also getting the same simplifying treatment.
Combining technology like industrial robots, manufacturing automation tools, and SICK sensors, all ends in the effect of opening vision technology to new users while allowing experienced users to improve their facilities and processes. It allows companies with fewer resources to automate effectively and efficiently, while giving experienced users another avenue for development and continuous improvement.
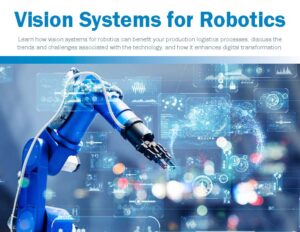
Download our ebook for more details on using machine vision for robotics.